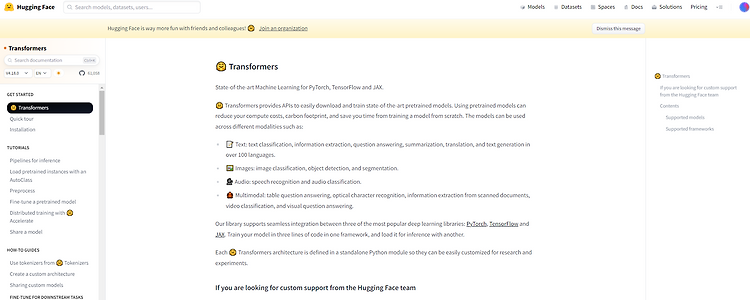
How to use "Hugging Face"(허깅페이스) for NLP Task
·
Artificial_Intelligence🤖/etc
허깅페이스는 Tensorflow Hub와 유사한 기능을 제공하는 곳이다. 트랜스포머를 기반으로 하는 다양한 모델들이 존재하며, 각각의 Task에 맞게 미세조정을 진행한 모델들 또한 구축되어있다. 또한, 데이터를 각 모델에 맞게 전처리하기 편리하도록 Tokenizer도 전부 구현되어있다. 그 뿐만 아니라, 학습을 위해 필요한 데이터셋도 저장되어 있어, 사용자는 그저 가져온 뒤 사용하기만 하면 된다. 즉, 허깅페이스를 사용하면 기존 학습 스크립트에서 반복되는 모든 부분을 일일이 따로 구현하지 않아도 편리하게 사용할 수 있으며, 데이터 구축부터 전처리, 모델 학습 및 결과 도출까지 매우 편리하고 효율적으로 코딩할 수 있다는 장점이 있다. 허깅페이스는 다양한 트랜스포머 모델과 학습 스크립트를 제공하는 모듈로, ..