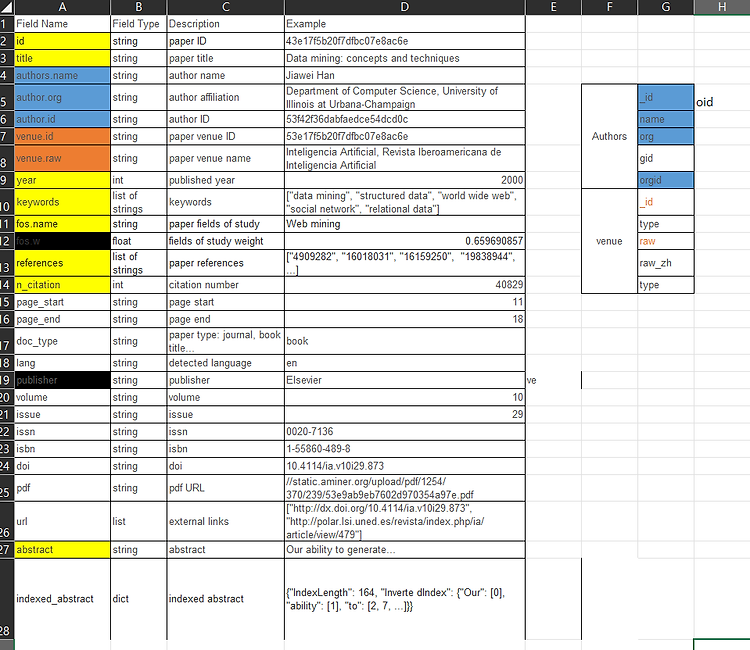
DBLP DataSet Processing / ๋์ฉ๋ Json ํ์ฑ
ยท
Artificial_Intelligence๐ค/Natural Language Processing
๊ทธ๋ํ ์๋ฒ ๋ฉ์ ๊ณต๋ถํ๊ธฐ ์ํ DataSet์ผ๋ก DBLP๋ก ์ ํ๊ณ ์ด๋ฅผ ๊ฐ์ ธ์๋ณด์๋ค. https://www.aminer.org/citation AMiner www.aminer.org ์ด ๊ณณ์ ๋ค์ด๊ฐ์ ์ด ๋ฐ์ดํฐ๋ฅผ ๊ฐ์ ธ์์ ๋ค์ด๋ก๋๋ฅผ ๋ฐ์๋ค. ๊ทธ๋ฐ๋ฐ ๋ฌธ์ ๋ ์ด ๋ฐ์ดํฐ๋ฅผ ๊ฐ์ ธ์์ ์ ์ฒ๋ฆฌ๋ฅผ ํด์ผํ๋๋ฐ ์ฉ๋์ด 16.1GB ์ด๋ค.. ์ฌ๋งํ ์๋ํฐ๋ก ์ด๋ฆฌ์ง๋ ์๋ ๋ฐ์ดํฐ๋ฅผ ์ฒ๋ฆฌํด์ผํด์ ๋ง๋งํ์๋ค. ๊ทธ๋์ ์๊ฐํ ๊ฒ์ด ๋ฐ์ดํฐ๋ฅผ ์ฉ๋์ ์ ํด์ ์๋ฅด๊ณ , ์๋ฅธ ์ฝ๋๋ฅผ ์์์
์ผ๋ก ์กฐ๊ธ๋ง ์๋ด์ฃผ์๊ณ ์๊ฐํ์๋ค. ๋ด๊ฐ ์ฌ์ฉํ ํ๋ก๊ทธ๋จ์ GSplit 3 ์ด๋ค. ์ฌ๊ธฐ์ ๊ฐ์ ธ์จ DBLP Jsonํ์ผ์ ๊ฐ์ ธ์์ 1GB์ฉ ๋จผ์ ์๋๋ค. ์ด๋ ๊ฒ ๋๋ฉด, ๋์
๋๋ฆฌ๋ก ์๋ฅด๋ ๊ฒ์ด ์๋ ์ฉ๋์ผ๋ก ์๋ฅด๊ธฐ์ Json ํ์์ด ๊นจ์ง๊ฒ ๋๋ค. ๋ฐ๋ผ์, ..